Beekeeping is an age-old practice that makes humans rely on honeybees for honey production and pollination. As per the latest research by Environment America Research & Policy Center, it has been estimated that humans rely on bees to pollinate 73 of the 103 crops that offer more than 90% of bee colonies. At primordial times, beekeepers used to monitor hive health and productivity through manual inspections. This technique is time consuming and solely reliant on manual observation. However, with the advancements in technology AI and computer vision models, beehive monitoring can be automated.Â
The modern technology is capable of analyzing hive health, detect signs of disease, classify species & pollen in real time by administering beekeepers with insights easily. Harnessing robust AI-driven monitoring, beekeepers can improve hive health and promote effective pollination in agricultural ecosystems. This blogpost focuses on the multiple ways in which beekeepers can improve colony productivity by integrating computer vision into beekeeping. The context sheds a huge light on the effective impact of the future of hive monitoring with computer vision.
Practical challenges in modern beekeeping
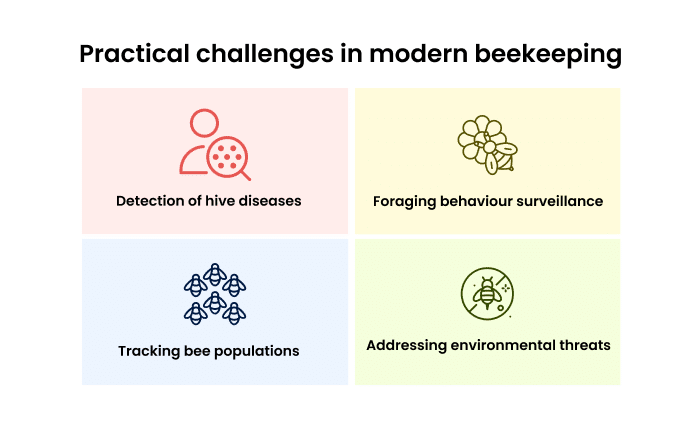
Beekeepers confront a huge variety of challenges when it comes to maintaining colony health and productivity. Traditional techniques are time-consuming and less efficient posing perils to sustain thriving hives.         Â
Detection of hive diseases
Several pests and bacterial infections tend to spread quickly within a beehive colony. Early detection of this infection or pests is valuable to maintaining a healthy hive colony.Â
Foraging behaviour surveillance
Learning about bees and the flowers they visit assists in optimizing pollination and tracking bee activity across large areas. This entire process can be challenging without automation.
Tracking bee populations
It is important to keep an accurate record of bees leaving and returning to the hive to understand colony strength. Manual counting is next to impossible.
Addressing environmental threats
Several environmental conditions, such as climate change, loss of habitat, and exposure to extreme temperature fluctuations, are potential risks that should be observed in real time.Â
Considering the above list of challenges, real-time monitoring and data-oriented solutions can help automate different tasks. It diminishes overall human workload and offers real-time monitoring.        Â
Practical applications of computer vision in beekeeping
In this context, we will take a closer look at AI-powered vision systems and how they can help beekeepers enhance smart hive monitoring and pollination tracking.Â
Bee early disease detection
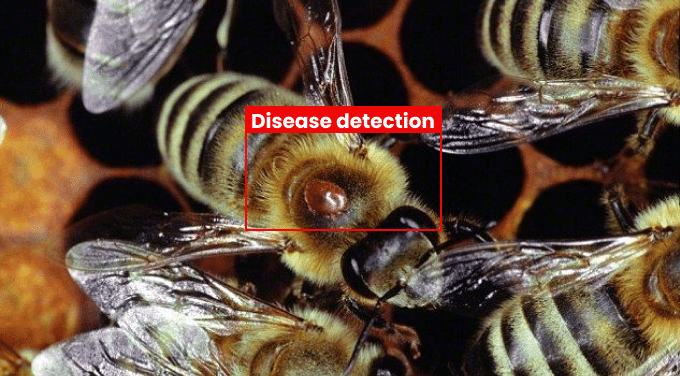
For beehive colonies, the most common parasite attack is the Varroa destructor. It weakens the bees and results in colony collapse disorder. Harnessing the object detection system significantly identifies mites early and prevents further infestations.
Flower detection & classification
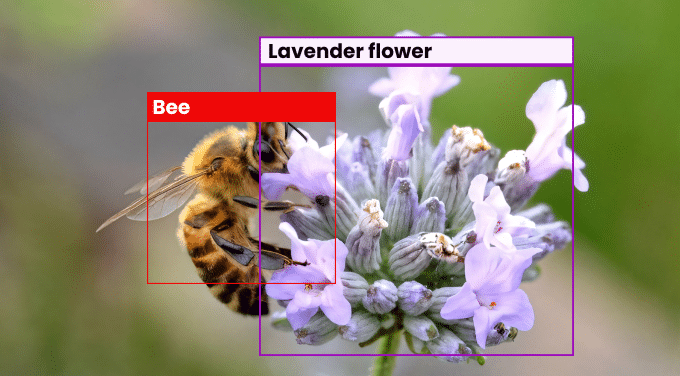
By detecting high-value nectar sources, beekeepers can make informed decisions that support both honey yield and colony wellbeing. Computer vision in beekeeping is trained in a way that can classify and detect distinct flower species by analyzing images captured by drones. This data can be used by beekeepers to maintain access to diverse floral resources, adjust hive placement, and support pollination for local agriculture.Â
Activity monitoring
For evaluating bee colony health, it is important to track bee populations. Sudden declines in activity can indicate food insufficiency, environmental stress, and disease. Manual supervision and counting of bees is not possible. Computer vision models are capable of analyzing real-time video from hive entrance cameras for counting the total number of bees. AI-based systems are capable of tracking different fluctuations in activity assisting beekeepers detect signs of colony stress. Computer vision models assist in identifying bees that have successfully pollinated flowers by identifying traces of pollen on their bodies.
Automated tasks
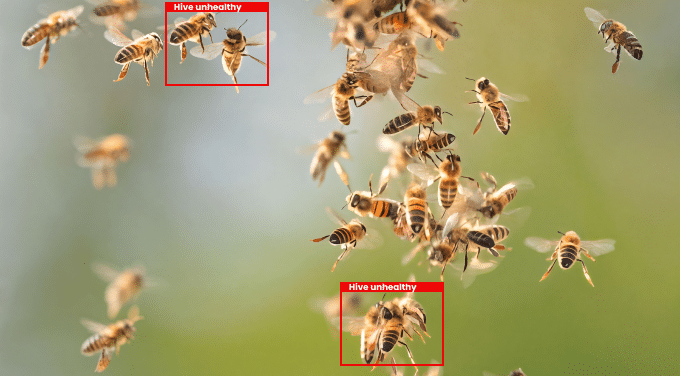
Leveraging AI models, computer vision helps in counting bees and analyzing frame images automating its techniques. The smart farming solutions and beehive AI monitoring system help in making data-driven decisions and maintaining hive health.
Remote monitoring
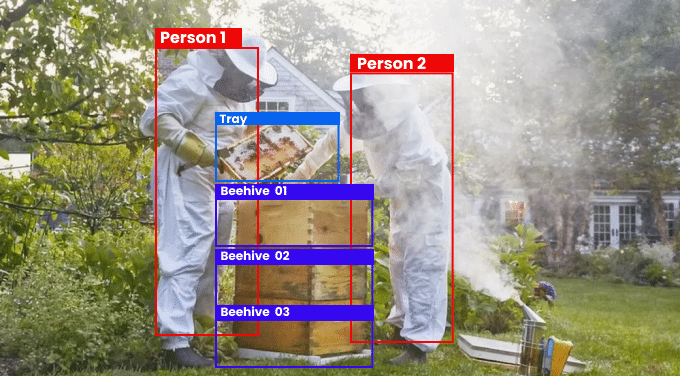
The real-time monitoring of computer vision models can seamlessly classify and detect distinct flower species by analyzing images captured through cameras. These cameras are equipped with an AI computer vision model, which provides real-time surveillance of beehive colonies.
Pollen type detection
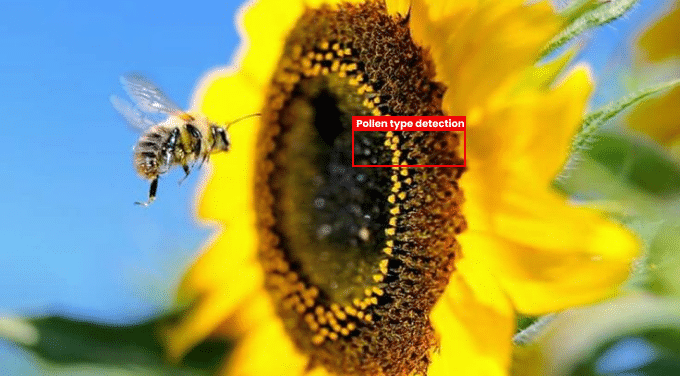
Beehive keepers can embrace a data-driven approach to beekeeping, analyzing the pollen gathered by bees can offer valuable insights into colony nutrition. Vision AI models can be trained on microscopic pollen images and can seamlessly differentiate pollen types depending on their unique structure. By analyzing pollen samples gathered by bees, AI-enabled computer vision models can determine which plant species are primarily contributing to the colony’s diet. With the effective integration of computer vision into hive monitoring, beekeepers can make more informed decisions regarding hive placement, honey processing and forage availability.Â
Process followed by computer vision to improve hive monitoring
Computer vision processes visual data from cameras and sensors for analyzing hive activity. The process involves tracking bee movement, classifying environmental factors, real-time monitoring of beehive colonies and detecting abnormalities. Vision AI systems provide beekeepers with deeper insights while reducing the disruption to hives. In order to integrate computer vision into hive monitoring, the below-mentioned steps can be followed.  Â
Data gathering
The process of collecting images and videos of the hive entrances, floral environments and bees.Â
Data annotation
It is important to label images for identifying pests, flowers, bees and pollen types.
Model training
It comprises training computer vision models on datasets for recognizing normal and abnormal bee activity, virus infestation, disease symptoms and preferred foraging sites. Â
Validation & testing
Model accuracy can be evaluated across different hive environments and lighting conditions.
Deploying monitoring systems
Computer vision systems powered by AI can be integrated to administer real-time analytics.Â
Following the above-mentioned steps computer vision models can be easily customized for detecting beehive environments. Harnessing Vision AI in agriculture, beekeepers can respond proactively to optimize management strategies.Â
Conclusion
With beekeeping facing new challenges, computer vision models can easily support beekeepers by automating early detection of diseases, hive monitoring, and optimizing pollination identification. Right from bee counting and disease detection to flower classification and pollen analysis, AI-powered solutions administer healthy colonies and improve honey production in the long run. As the leading computer vision development company, Nextbrain provides AI Video analytics software for different businesses. The software is designed in a way tailor-made for businesses that can distinguish between bees carrying pollen and returning to the hive without it.Â
Do you want to know more about beekeeping powered by an AI computer vision model? Get in touch with our professionals to learn more about AI video analysis.